Commentary: Despite continued advances in AI, we still haven't solved some of its most basic problems.
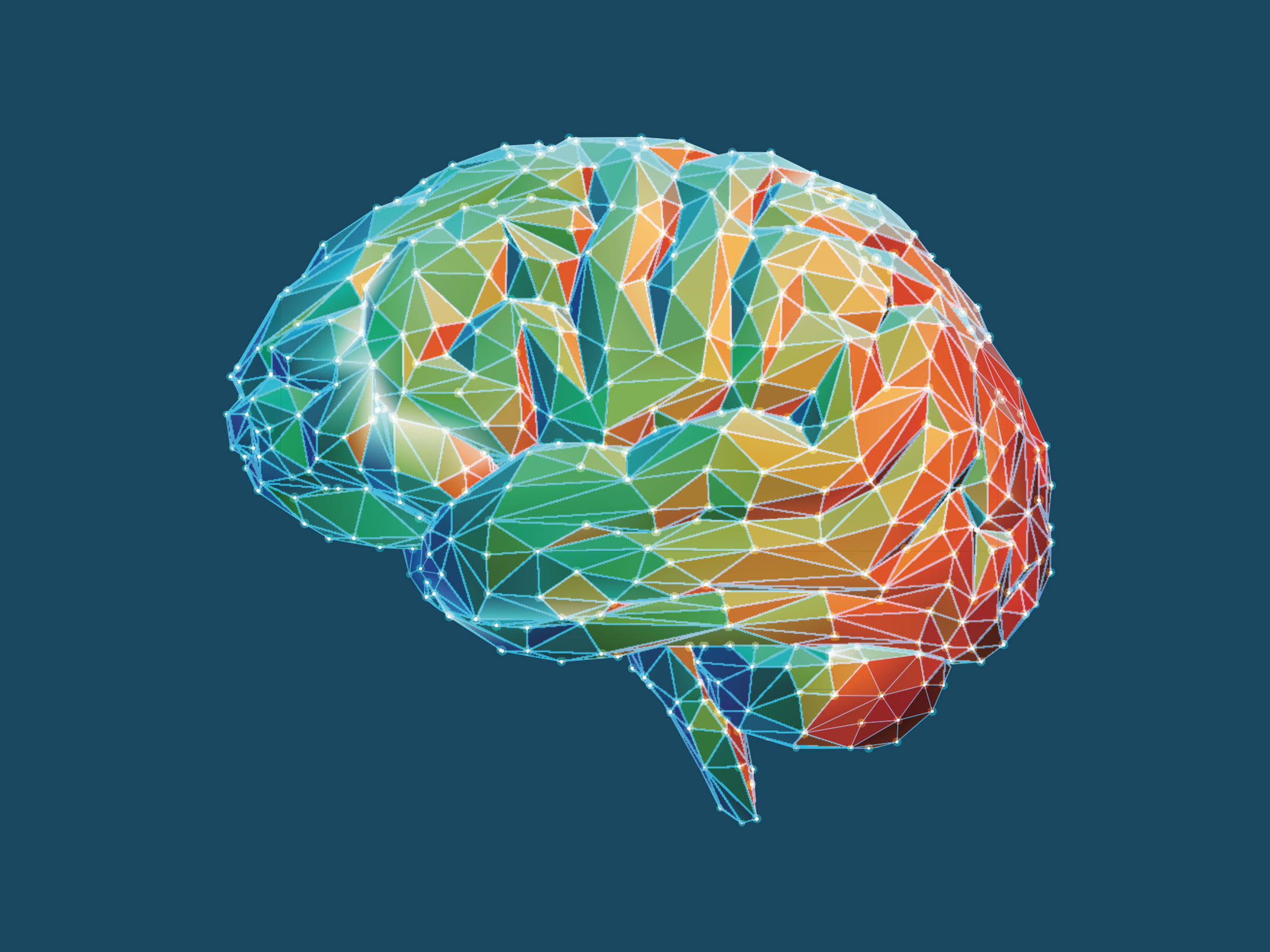
Image: iStock/Jolygon
We've been so worried about whether AI-driven robots will take our jobs that we forgot to ask a much more basic question: will they take our bike lanes?
That's the question Austin, Texas, is currently grappling with, and it points to all sorts of unresolved issues related to AI and robots. The biggest of those? As revealed in Anaconda's State of Data Science 2021 report, the biggest concern data scientists have with AI today is the possibility, even likelihood, of bias in the algorithms.
SEE: Artificial intelligence ethics policy (TechRepublic Premium)
Move over, robot
Leave it to Austin (tagline: "Keep Austin weird") to be the first to have to grapple with robot overlords taking over their bike lanes. If a robot that looks like a "futuristic ice cream truck" in your lane seems innocuous, consider what Jake Boone, vice-chair of Austin's Bicycle Advisory Council, has to say: "What if in two years we have several hundred of these on the road?"
If this seems unlikely, consider just how fast electric scooters took over many cities.
The problem, then, isn't really one of a group of Luddite bicyclists trying to hammer away progress. Many of them recognize that one more robotic delivery vehicle is one less car on the road. The robots, in other words, promise to alleviate traffic and improve air quality. Even so, such benefits have to be weighed against the negatives, including clogged bike lanes in a city where infrastructure is already stretched. (If you've not been in Austin traffic recently, well, it's not pleasant.)
As a society, we haven't had to grapple with issues like this. Not yet. But if "weird" Austin is any indicator, we're about to have to think carefully about how we want to embrace AI and robots. And we're already late in coming to grips with a much bigger issue than bike lanes: bias.
Making algorithms fair
People struggle with bias, so it's not surprising the algorithms we write do, too (a problem that has persisted for years). In fact, ask 3,104 data scientists (as Anaconda did) to name the biggest problem in AI today, and they'll tell you it's bias (Figure A).
Figure A

Image: Anaconda
That bias creeps into the data we choose to collect (and keep), as well as the models we deploy. Fortunately, we recognize the problem. Now what are we doing about it?
Today, just 10% of survey respondents said their organizations have already implemented a solution to improve fairness and limit bias. Still, it's a positive sign that 30% plan to do so within the next 12 months, compared to just 23% in 2020. At the same time, 31% of respondents said they don't currently have plans to ensure model explainability and interpretability (which permeability would help to mitigate against bias), 41% said they've already started to work on doing so, or plan to do so within the next 12 months.
So, are we there yet? No. We still have lots of work to do on bias in AI, just as we need to figure out more pedestrian topics like traffic in bike lanes (or fault in car accidents involving self-driving cars). The good news? As an industry, we're aware of the problem and increasingly working to fix it.
Disclosure: I work for AWS, but the views expressed herein are mine.
Data, Analytics and AI Newsletter
Learn the latest news and best practices about data science, big data analytics, and artificial intelligence. Delivered Mondays
Sign up todayAlso see
Why machine learning, not artificial intelligence, is the right way forward for data science (TechRepublic)
Behind the scenes: A day in the life of a data scientist (TechRepublic)
Big data's role in COVID-19 (free PDF) (TechRepublic)
Data Encryption Policy (TechRepublic Premium)
Big data: More must-read coverage (TechRepublic on Flipboard)